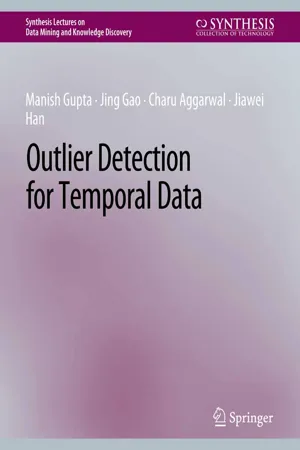
Outlier Detection for Temporal Data
Manish Gupta, Jing Gao, Charu Aggarwal, Jiawei Han
- English
- PDF
- Disponibile su iOS e Android
Outlier Detection for Temporal Data
Manish Gupta, Jing Gao, Charu Aggarwal, Jiawei Han
Informazioni sul libro
Outlier (or anomaly) detection is a very broad field which has been studied in the context of a large number of research areas like statistics, data mining, sensor networks, environmental science, distributed systems, spatio-temporal mining, etc. Initial research in outlier detection focused on time series-based outliers (in statistics). Since then, outlier detection has been studied on a large variety of data types including high-dimensional data, uncertain data, stream data, network data, time series data, spatial data, and spatio-temporal data. While there have been many tutorials and surveys for general outlier detection, we focus on outlier detection for temporal data in this book. A large number of applications generate temporal datasets. For example, in our everyday life, various kinds of records like credit, personnel, financial, judicial, medical, etc., are all temporal. This stresses the need for an organized and detailed study of outliers with respect to such temporal data. In the past decade, there has been a lot of research on various forms of temporal data including consecutive data snapshots, series of data snapshots and data streams. Besides the initial work on time series, researchers have focused on rich forms of data including multiple data streams, spatio-temporal data, network data, community distribution data, etc. Compared to general outlier detection, techniques for temporal outlier detection are very different. In this book, we will present an organized picture of both recent and past research in temporal outlier detection. We start with the basics and then ramp up the reader to the main ideas in state-of-the-art outlier detection techniques. We motivate the importance of temporal outlier detection and brief the challenges beyond usual outlier detection. Then, we list down a taxonomy of proposed techniques for temporal outlier detection. Such techniques broadly include statistical techniques (like AR models, Markov models, histograms, neural networks), distance- and density-based approaches, grouping-based approaches (clustering, community detection), network-based approaches, and spatio-temporal outlier detection approaches. We summarize by presenting a wide collection of applications where temporal outlier detection techniques have been applied to discover interesting outliers. Table of Contents: Preface / Acknowledgments / Figure Credits / Introduction and Challenges / Outlier Detection for Time Series and Data Sequences / Outlier Detection for Data Streams / Outlier Detection for Distributed Data Streams / Outlier Detection for Spatio-Temporal Data / Outlier Detection for Temporal Network Data / Applications of Outlier Detection for Temporal Data / Conclusions and Research Directions / Bibliography / Authors' Biographies